20 Unwritten Rules About Machine Learning
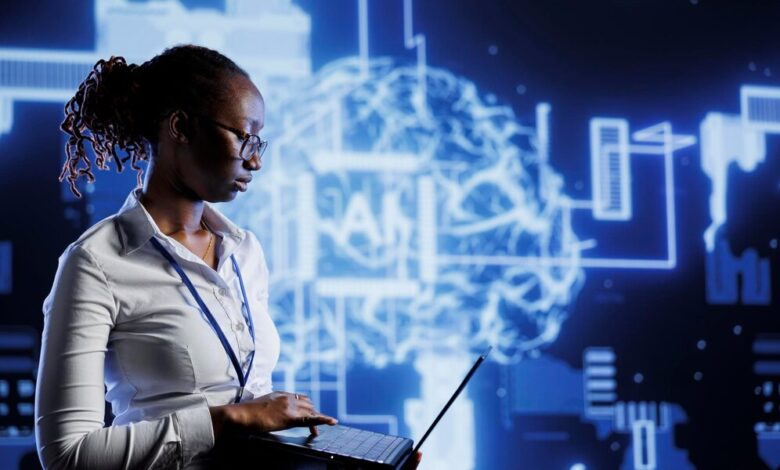
Machine learning has become one of the most exciting and transformative fields in technology today. Yet, beyond the textbooks and online courses, there are countless lessons that only experience can teach. Whether you’re a beginner or a seasoned practitioner, understanding these “unwritten rules” can save you time, effort, and a lot of frustration. Here are 20 essential unwritten rules about machine learning that everyone should know.
1. Your Data Matters More Than Your Algorithm
No matter how fancy your model is, if your data is poor quality, your results will be poor too. Clean, rich, and relevant data often beats complex models.
2. Simple Models First
Always start with the simplest model that could possibly work. You’ll be surprised how often a basic linear model performs remarkably well.
3. Overfitting Happens Sooner Than You Think
If your model is performing perfectly on training data, it’s probably overfitting. Always watch out for that early sign.
4. Feature Engineering Is Half the Battle
Choosing the right features can make or break your model. Sometimes, better features outperform better algorithms.
5. You Will Underestimate Data Preprocessing Time
Preparing your data—handling missing values, normalization, encoding—often takes far more time than building the model itself.
6. Not All Metrics Are Equal
Accuracy isn’t always the best metric, especially in imbalanced datasets. Precision, recall, F1-score, and AUC can often tell a deeper story.
7. There’s No “Best” Algorithm
No single machine learning algorithm works best for every problem. Testing and comparison are essential.
8. Bias in, Bias out
If your training data is biased, your model will be too. Be mindful of societal biases hidden in your datasets.
9. Interpretability Matters
Sometimes a simpler, more interpretable model is more valuable than a complex “black box” model, especially in critical sectors like healthcare and finance.
10. Tuning Hyperparameters Can Be a Black Hole
Hyperparameter optimization is important, but it can consume infinite time if you’re not strategic. Start simple before going deep.
11. Garbage Results Are Usually Your Fault
Before blaming libraries, frameworks, or algorithms, check your data and assumptions first. Most issues arise from user errors.
12. Default Settings Are Not Always Best
Libraries like Scikit-learn or TensorFlow provide default settings, but they are not optimized for your specific problem.
13. Beware of Data Leakage
Using information from outside your training data that wouldn’t be available at prediction time can give you an unfair advantage—and lead to failure in the real world.
14. Cross-Validation Is Your Friend
Don’t just rely on a single train/test split. Cross-validation helps in estimating the real performance of your model more reliably.
15. More Data Beats More Tweaks
Whenever possible, collecting more high-quality data usually improves your model more than endless tweaking.
16. Models Degrade Over Time
In production, models often become less accurate over time due to changes in real-world conditions. Monitoring and retraining are essential.
17. Explain Your Results to Non-Experts
If you can’t explain your model’s behavior to a non-technical audience, you’ve lost half the battle. Communication is key.
18. Reproducibility Is Non-Negotiable
You must be able to recreate your results. Proper documentation, version control, and random seed settings are crucial.
19. Frameworks Change, Fundamentals Stay
Today it’s PyTorch and TensorFlow; tomorrow it could be something else. But concepts like gradient descent and regularization will still be fundamental.
20. Stay Humble
Machine learning is a vast and rapidly changing field. No matter how much you know, there’s always more to learn—and that’s what makes it exciting.
Mastering machine learning isn’t just about coding up models; it’s about developing an instinct for what works and what doesn’t. These unwritten rules reflect lessons that many professionals learn the hard way. Keep them in mind as you grow in your machine learning journey, and you’ll be ahead of the curve.